2024 AIChE Annual Meeting
(673a) High-Throughput Virtual Screening and Hybrid Computational/Experimental Active Learning for Novel Hydrogel-Nanoparticle Sensors for Fentanyl Detection
Authors
Shayesteh Zadeh, A. - Presenter, University of Illinois Urbana-Champaign
Winton, A., Army Research Laboratory
Coppock, M., Army Research Laboratory
Palomba, J. M., U.S. Army DEVCOM Soldier Center
Ferguson, A., University of Chicago
Fast, portable, and reliable detection of toxic chemical and biological compounds is an important challenge in many security and healthcare applications. Chemical sensors based on photonic crystals that use protein-catalyzed capture (PCC) agents as receptors are promising tools for on-the-fly detection of chemical and biological compounds. We report the development and deployment of a high-throughput screen utilizing experimental and computational data to discover PCC agent candidates that maximize binding affinity and specificity for fentanyl detection. We employ a multi-fidelity, multi-objective Bayesian optimization (MFMOBO) approach capable of asynchronous ingestion of computational predictions and experimental measurements to efficiently search the PCC design space for candidates that maximize fentanyl binding affinity and binding specificity relative to a decoy molecule. The MFMOBO approach performs guided sampling of a small fraction of chemical space to efficiently resolve the affinity-specificity Pareto frontier and identify the top-performing PCC candidates for incorporation into next-generation chemical sensing devices.
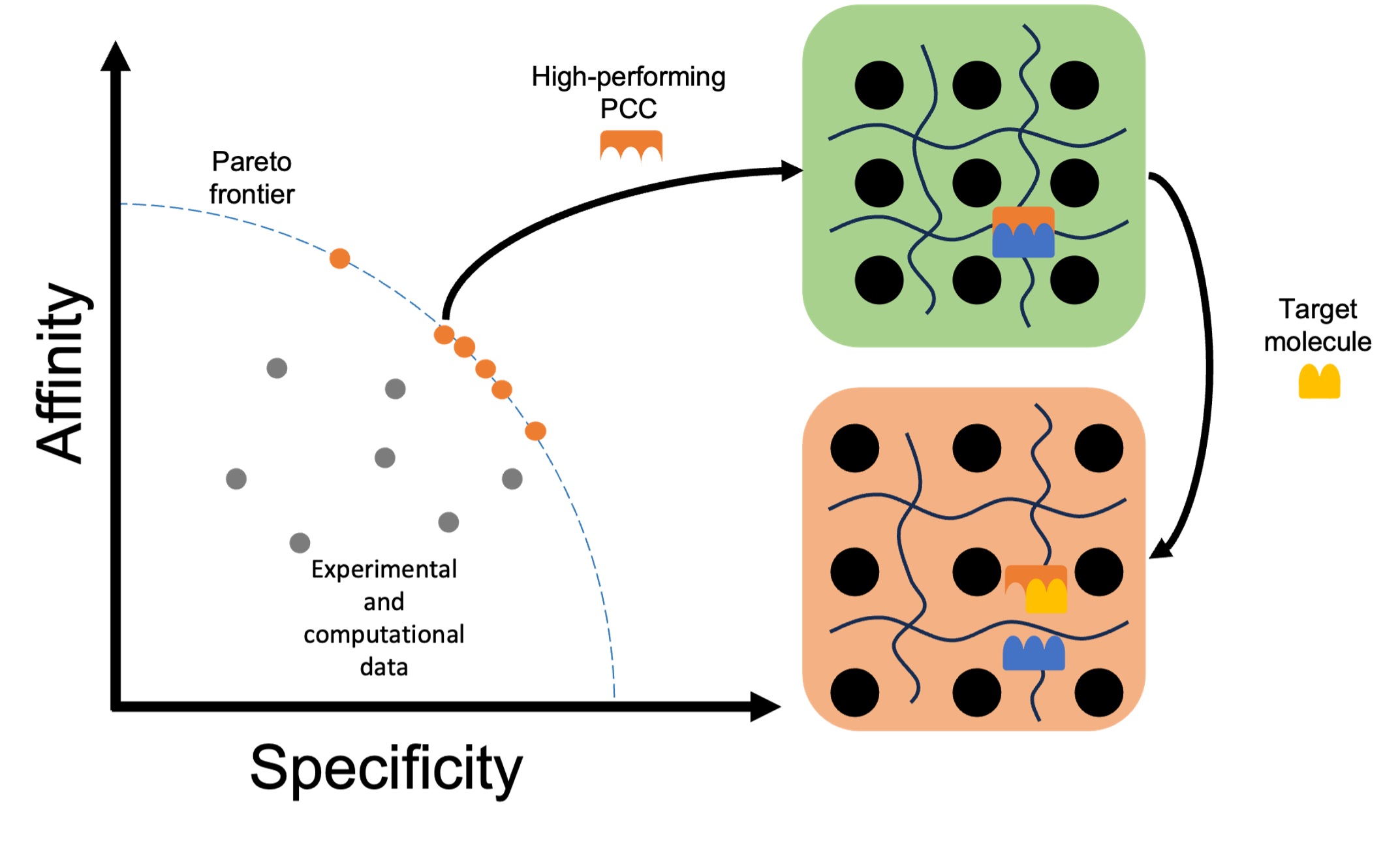
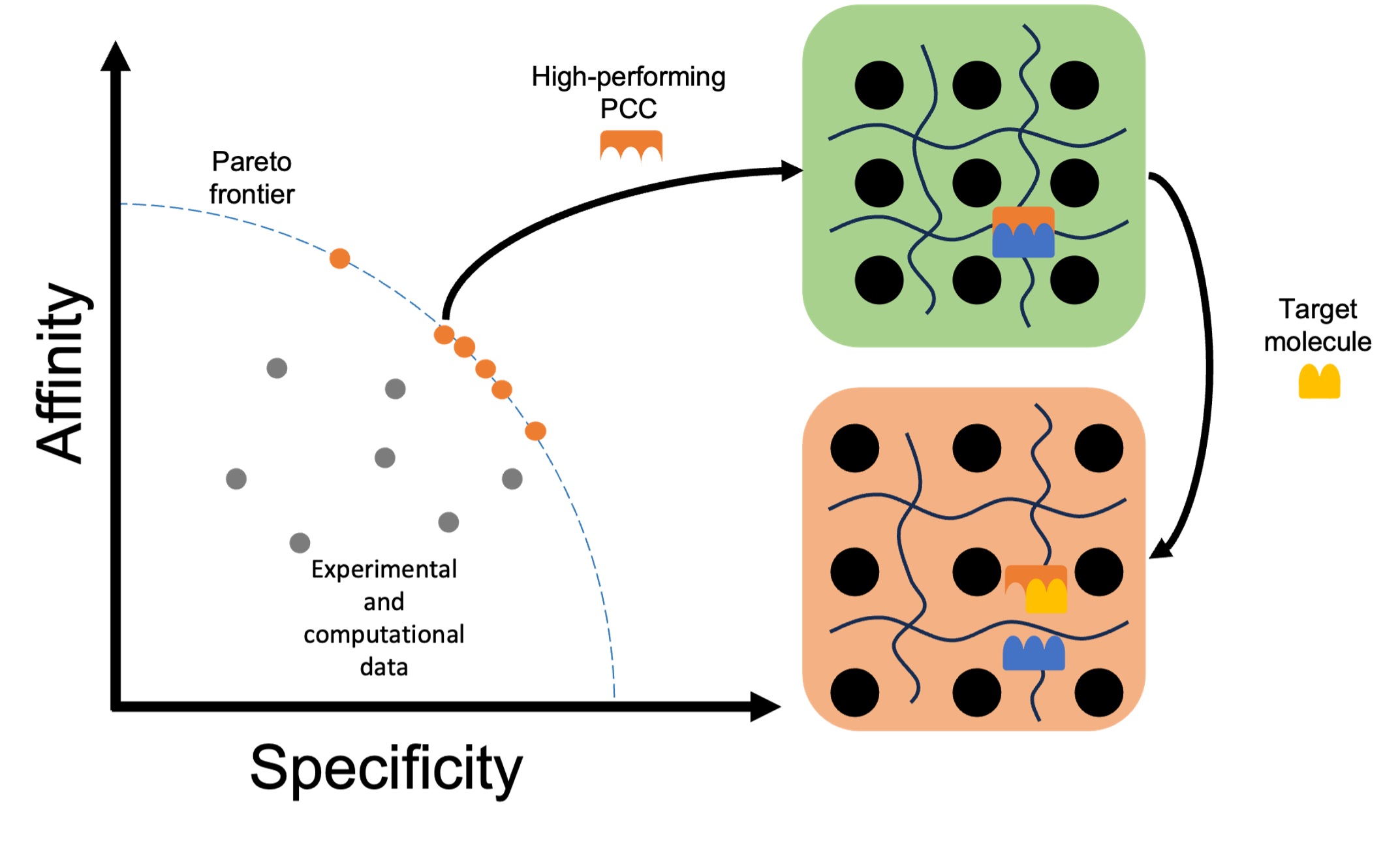