2024 AIChE Annual Meeting
(455e) Catberta: Catalyst Energy Prediction and Feature Analysis through Language Models
Effective screening of catalysts requires models that can predict adsorption energy, a crucial indicator of reactivity. Current prevailing methodologies, graph neural networks (GNNs), require precise atomic coordinates to build graph representations and struggle to incorporate observable characteristics in a human-interpretable way. This study introduces CatBERTa, a Transformer-based energy prediction model leveraging the pre-trained RoBERTa natural language processing encoder. CatBERTa directly processes human-readable text, including compositions and structural descriptions of catalysts. This text can be formatted as either strings containing specific features or as natural language descriptions produced by generative language models such as ChatGPT. CatBERTa's predictions of adsorption energy, based on textual representations of initial structures, match the accuracy of conventional GNNs, such as SchNet and DimeNet++. Notably, in data subsets where GNNs achieve high prediction accuracy, CatBERTa consistently exhibits comparable accuracy, with a mean absolute error (MAE) of 0.35 eV. An ablation study demonstrates the importance of interacting atoms as descriptors for adsorption configurations, while deeming bond lengths and atomic properties less predictive. Moreover, the self-attention score from the Transformer architecture enables us to understand the model's focus on tokens related to adsorbates, bulk composition, and interacting atoms. Analysis of the self-attention scores and latent space demonstrates that the fine-tuning process shifts the model's focus towards text pertaining to the adsorbates and adsorption configurations. This work lays the groundwork for text-based prediction of catalyst properties, bypassing the need for graph representations and shedding light on complex feature-property relationships.
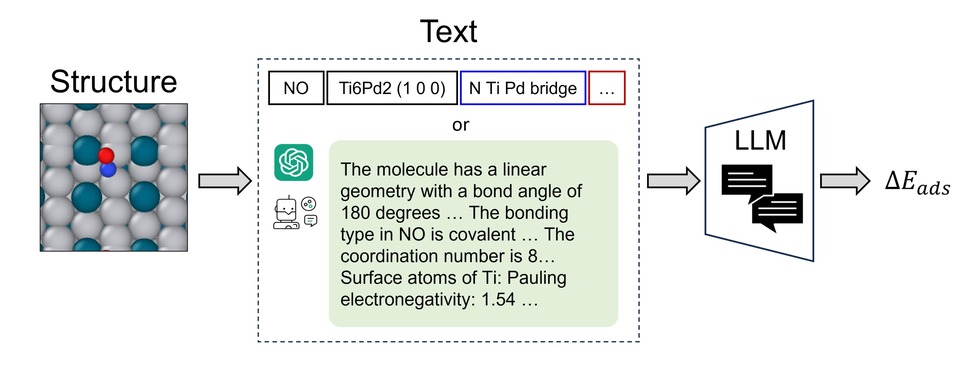
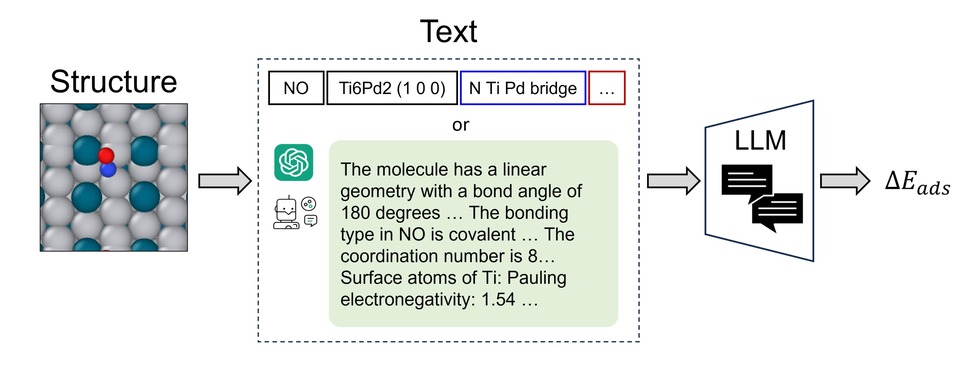