Generative artificial intelligence (AI) approaches are currently transforming various sectors. For instance, ChatGPT demonstrates impressive zero-shot performance for language generation. Likewise GitHub Copilot generates code, DeepL translates texts, Grammarly corrects texts, IBM RXN generates reaction outcomes, and Adobe Firefly generates images. The goal of generative AI models is to learn a parametric approximation of an underlying (unknown) data distribution. This allows to sample new data from the generative model mimicking the original data distribution. This technology is driven by a combination of big data, meaningful information representation, GPU computing, and modern deep learning architectures like transformers used in large language models. Besides the technology push, we are also in a situation with an exceptionally strong market pull. Big technology companies are investing heavily in generative AI as a considerable business potential is anticipated. Generative AI is believed to transform sectors and unleash the next wave of productivity. For example, McKinsey estimated in June 2023 that generative AI and other technologies have the potential to automate work activities that absorb 60 to 70 percent of employees’ time today. However, there is still limited application of generative AI in chemical process engineering.
We propose the first Large Language Model (LLM) for chemical process engineering. The model is called PILOT which stands for “Process Intelligence Learning and Optimization Tool”. We generate a training data set with common dialogs related to chemical process engineering. Then, we finetune an open source LLM architecture. Finally, we benchmark the performance and compare to existing open-source and commercial alternatives. We demonstrate the potential of our proposed LLM to design economically viable flowsheets in an illustrative case study. The presented concept is a significant step towards adaption of generative AI in the chemical process AI domain.
References
[1] Schweidtmann, A. M. (2024). Generative artificial intelligence in chemical engineering. Nature Chemical Engineering, 1(3), 193-193.
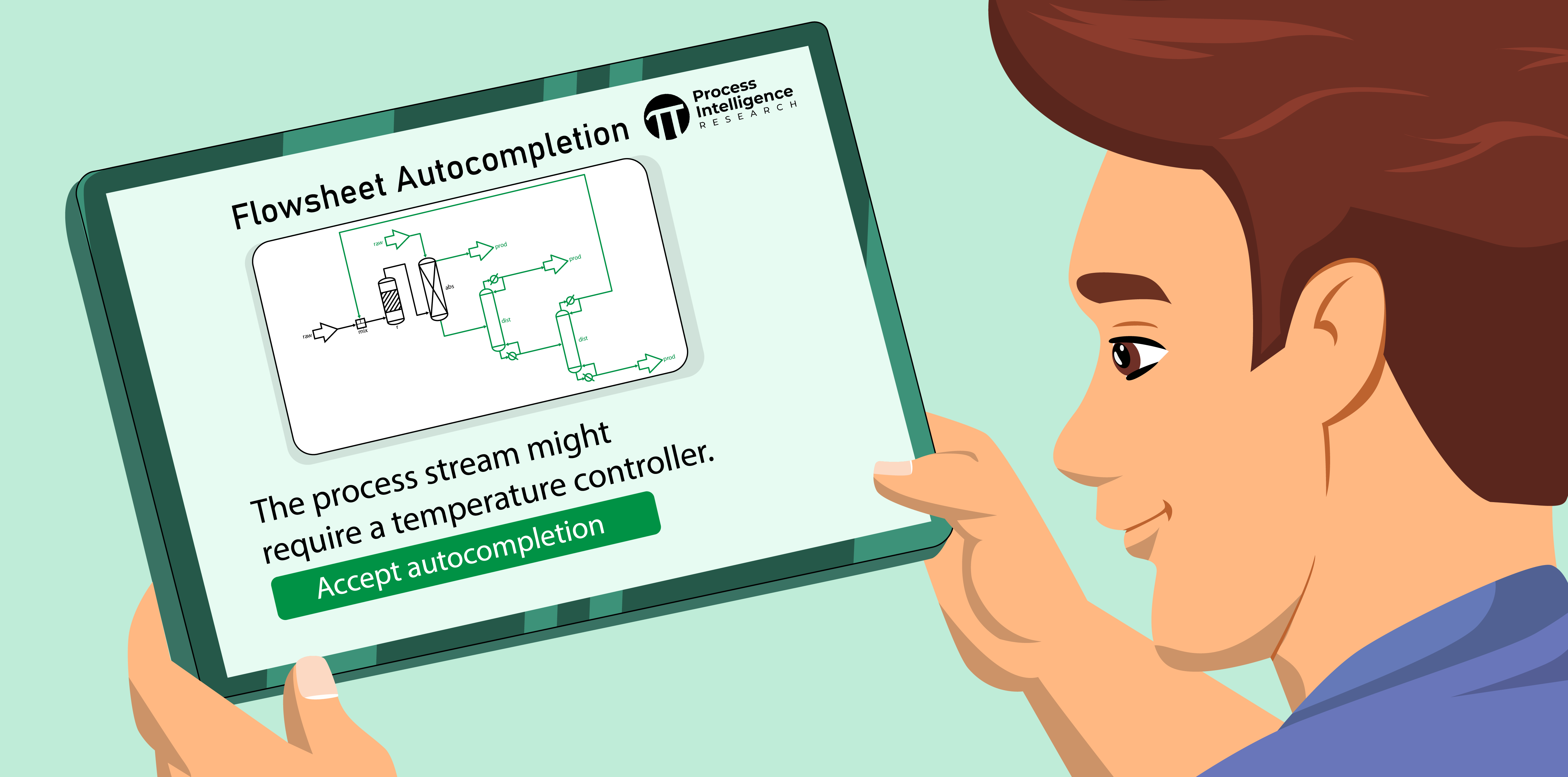