2022 Annual Meeting
(173f) Demonstrating the Affect of Polymer Type on Oil Yield in Thermal Depolymerization Using Machine Learning
Chemical recycling of plastics into fuels via processes such as pyrolysis and hydrothermal liquefaction (HTL) is a potentially viable way to handle the millions of tons of waste plastic generated globally. Thermal depolymerization reactions, especially in the presence of mixed plastic feeds, are comprised of complex reaction networks that make traditional physic-based modeling immensely difficult. Data-driven models, with careful model validation and testing, can provide an alternative to the traditional approach. In this study, a dataset consisting of 370 data points of both pyrolysis and HTL of plastic feeds was collected from the open literature. The data was first evaluated for pyrolysis conditions only, resulting in a dataset size of 310 data points. The dataset was divided into training and test sub-datasets and used to evaluate the performance of 7 different machine learning algorithms. Of these models Random Forest Regression and eXtreme Gradient Boosting (XGBoost) predicted the oil yield of the test set with the highest accuracy. They predicted the oil yield for this never-before-seen data with mean absolute error (MAE) values of 8.3% (Random Forest) and 8.7% (XGBoost), respectively. Using the same procedure, the whole dataset (including HTL data) was re-evaluated with the finding that the MAE corresponding to the Random Forest model was 8.5%, similar to that observed for pyrolysis data on its own. The optimized Random Forest model was then used to analyze the oil yield benefit of removing dense (and potentially problematic) plastics, such as polyethylene terephthalate (PET) and polyvinyl chloride (PVC), from pyrolysis feeds. Figure 1 shows the negative impact these polymers have on oil yields in various plastic mixtures.
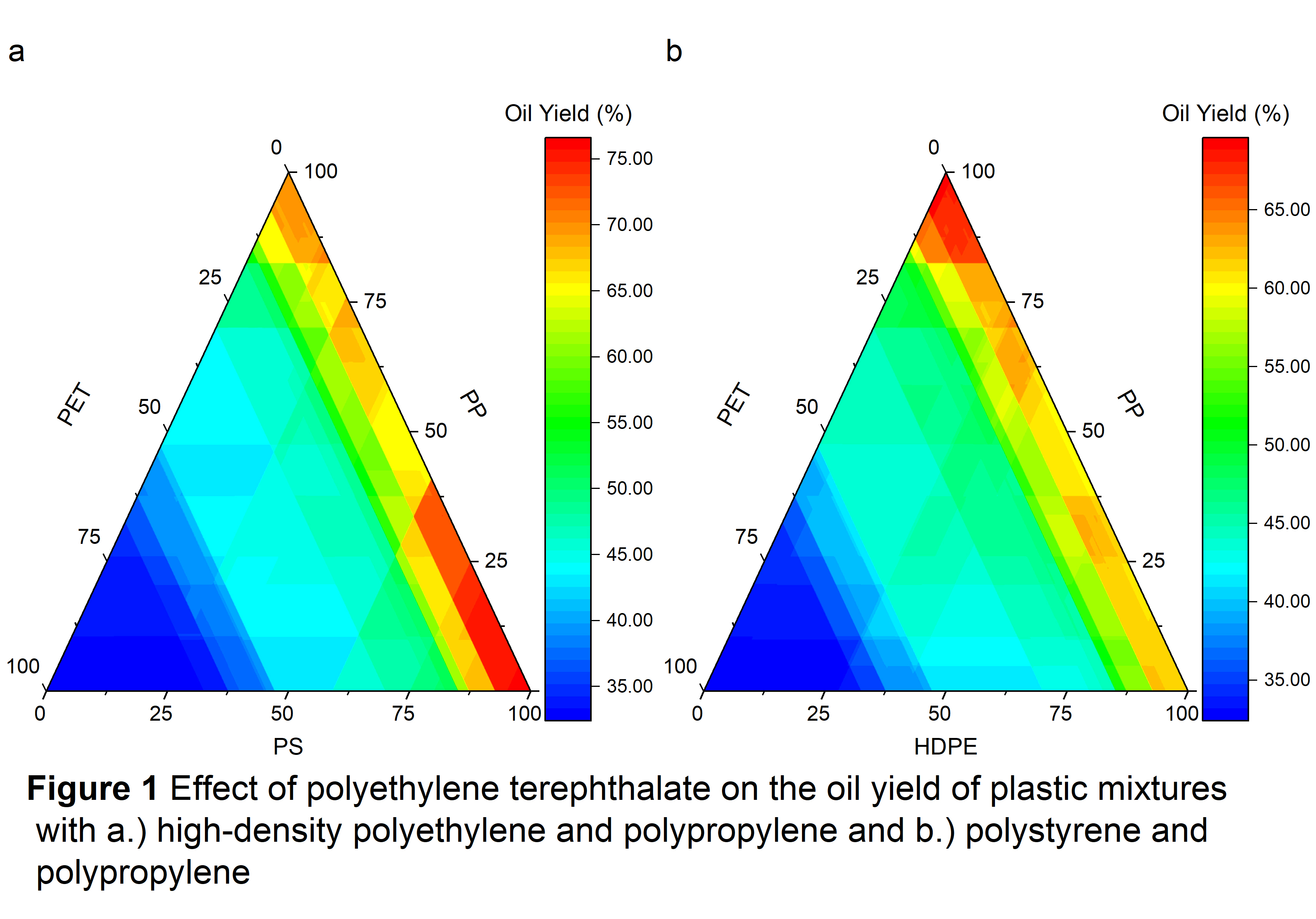
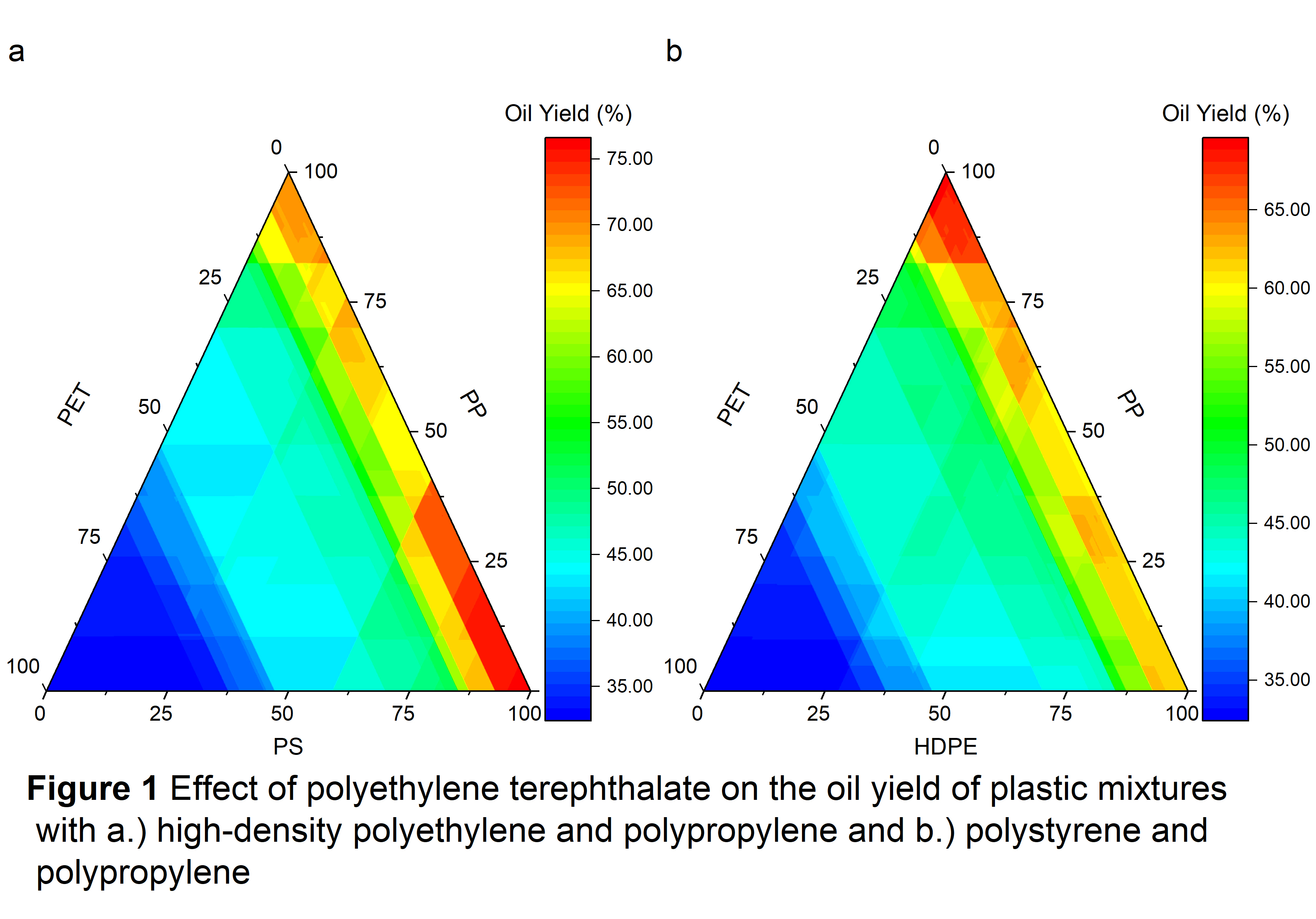