2020 Virtual AIChE Annual Meeting
(163r) Machine Learning Driven Insights into Defects of Zirconium Metal-Organic Frameworks for Enhanced Ethane-Ethylene Separation
Authors
Ying Wu - Presenter, South China University of Technology
Haipeng Duan, South China University of Technology
Hongxia Xi, South China University of Technology
Structural defects in metal-organic frameworks (MOFs) have potential to bring desirable properties that could not be achieved by âdefect-freeâ crystals, but previous works in this area have focused on limited versions of defects due to the difficulty of detecting defects in MOFs. In this work, a modeling library of 425 UiO-66-Ds with a broad population (in terms of concentration and distribution) of missing-linker defects was created, followed by the applications to ethane/ethylene separation. We demonstrated that machine learning could be an efficient and âsimulation-freeâ way to elucidate the key features governing the target properties (adsorption, separation and mechanical stability). Fully exploring the library by machine learning also allowed to capture some vital implications. Controlling defect concentration rather than distribution was found to be of more significance in tuning the overall properties. The missing-linker ratio in real UiO-66-D materials could be predicted from only gravimetric surface area and pore volume. This finding is extremely interesting, as it is expected to be a complementary solution towards the challenges of measuring the defect concentration in experiment. Finally, we provided decision trees as straightforward guidance to access the âprivilegedâ UiO-66-Ds with optimal overall properties, or to avid the poor candidates. Despite detection of defects in MOFs remains challenging experimentally, this work represents a significant step towards a data-driven analysis of MOF defects, and provide a useful reference for the development of defect-engineering in MOFs and related materials referring to practical adsorption/separation processes.
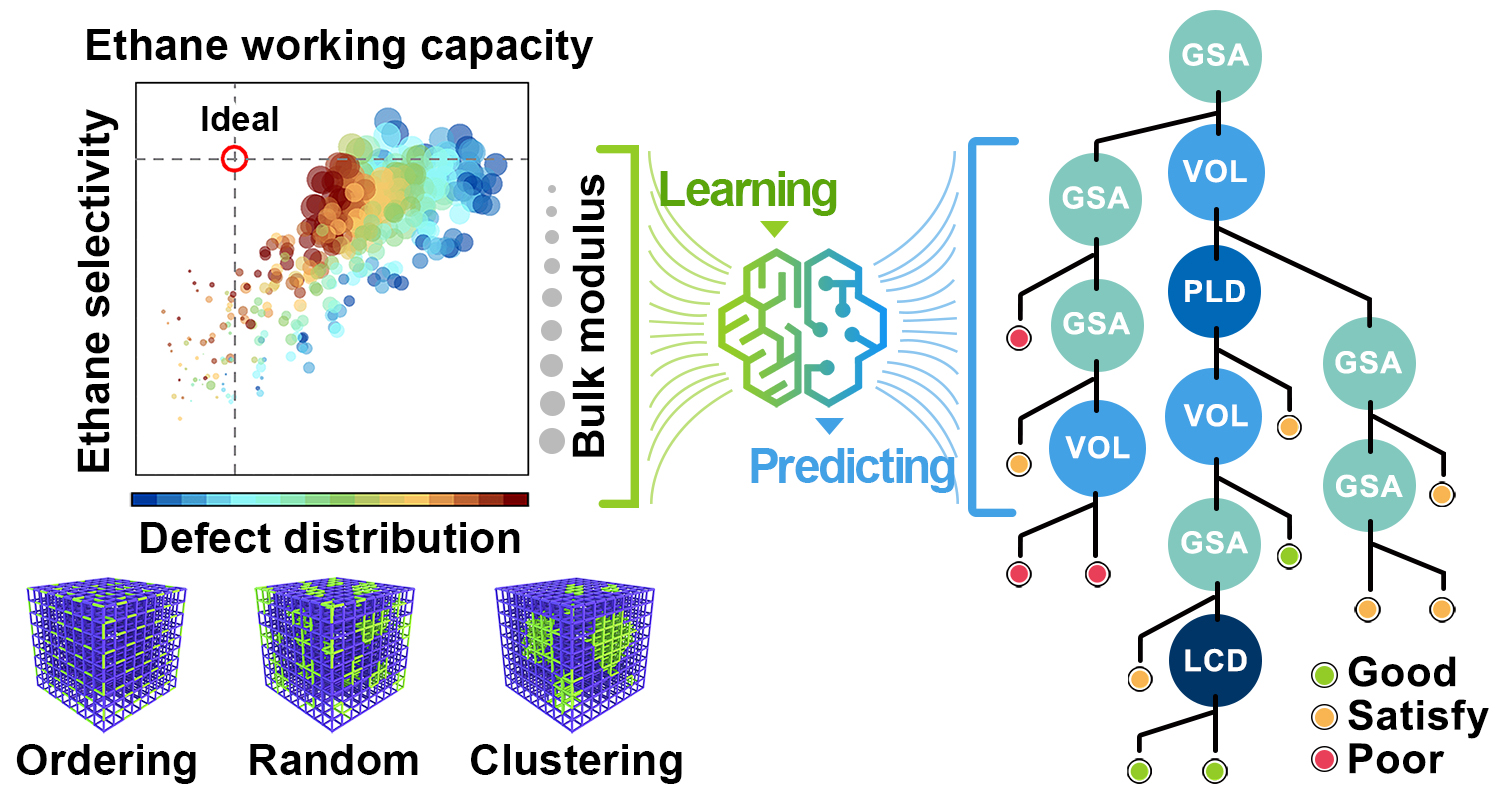
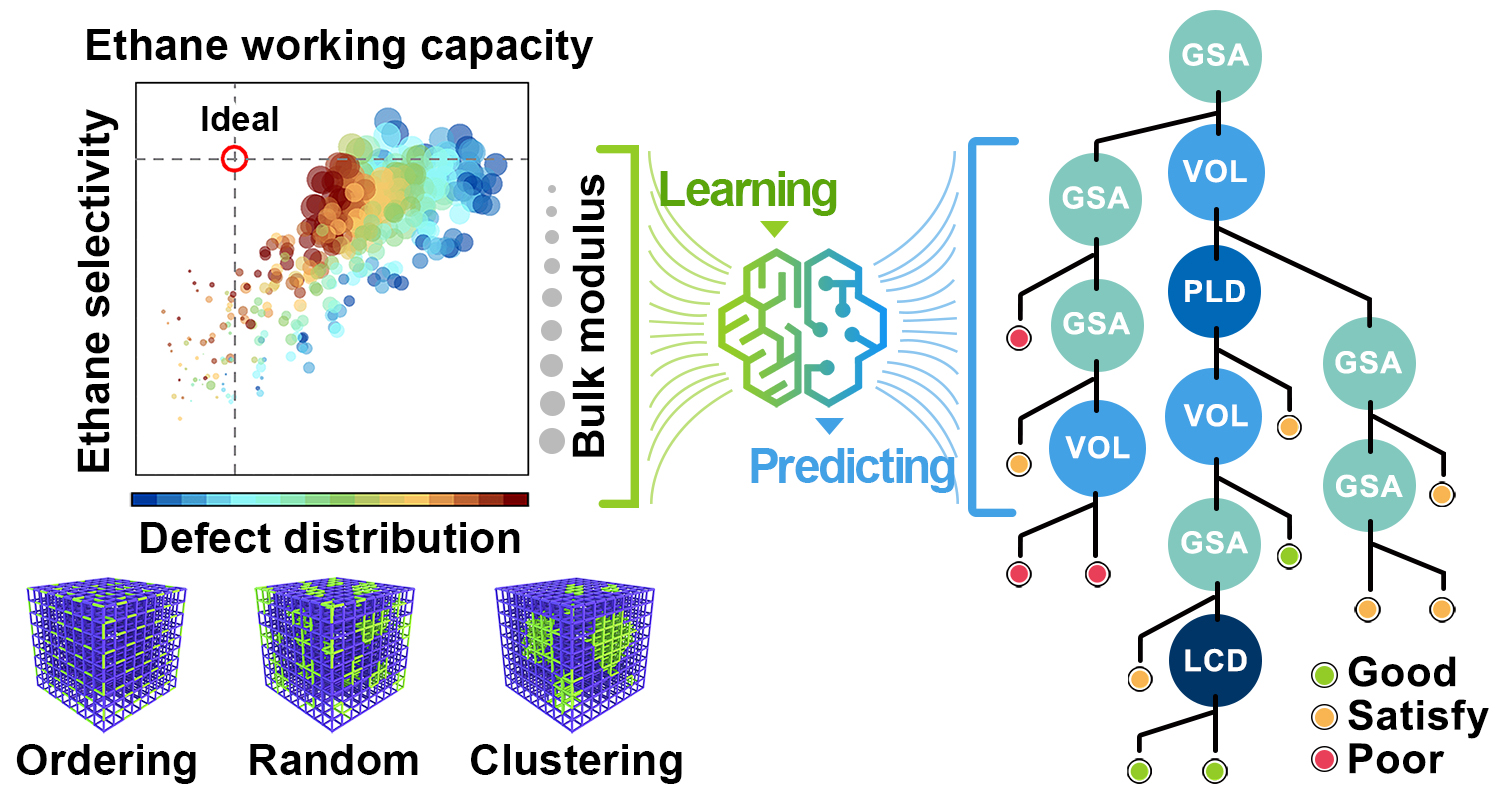